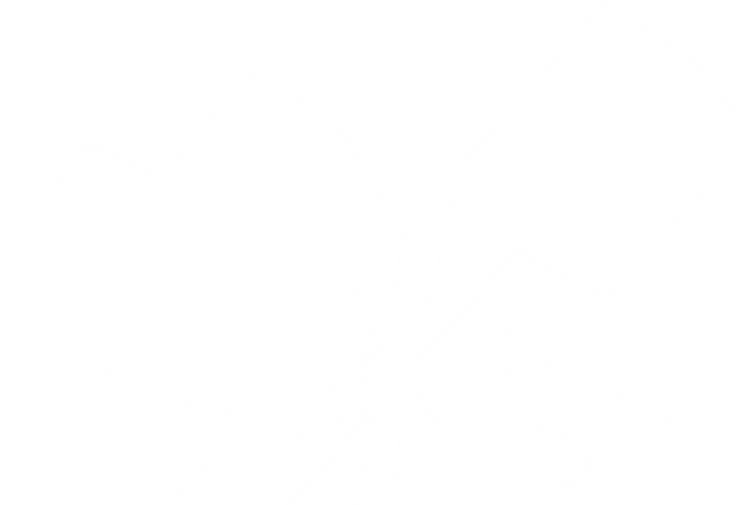
#04 Unveiling AI’s Role in Partner Marketing | with Rob Berrisford
Warm welcome to today's Partner Marketing Podcast. Everybody speaks about AI and everybody thinks about how to use it in business context. Where are we with AI in partner marketing? Which use cases do already exist? And how do I get started using AI for my own business? In today's episode, Rob Beresford, founder of Affiliate. ai, will share insights and best practices with us. Let's get started. Welcome to the Partner Marketing Podcast.
Hello, Rob. Warm welcome to the Partner Marketing Podcast. Thanks for having me. We will be speaking about AI. We will be speaking about partner marketing. And I think a bit more specifically, we will be speaking about AI in partner marketing. What would you say, what is the status of AI in partner marketing today? Almost non-existent. I would say. I think a lot of people are starting to experiment. So, you would say it's a little bit the same as in all other industries? Or maybe we are a little bit ahead? I think the experimentation in AI, which is largely where the sector is right now, is limited to the really big guys, the frontier model providers, and a whole bunch of startups who are like testing and learning what to do.
I think when we started Affiliate. AI, we kind of thought there'd be a rapid arms race in partner marketing to use this technology. But we've seen a lot of the networks and a lot of the agencies experimenting in the space, I would say. But very little has made it to customers, to trust the people that connect with them. So there's been some really negative stuff coming out of it. I think there's this idea of it being more... It's trend anymore. I've become more conversant in the handheld marketing industry than I was in the tech space, running this place. So, it's more about what tools do we have? So that's exactly the whole thing, that's a few bits there.
There's like you know, the killer use case, I think across all of um AI people are still hunting for like we thought that that chat would be the new front end for the internet and and you know websites would be gone and search would be gone and candidly, we we're not seeing that. People necessarily want to chat with things in the same way that uh we expected them to. And so, you know, a lot of AI is still looking for that killer use case um. We still have the question of accuracy in AI; I call it kind of you know people refer to it as hallucinations, but you know these are probabilistic systems rather than deterministic systems.
Which is how traditional software is built, and so you do get inconsistent results um which is fine when you're asking ChatGPT to write a poem about you know two people on a podcast but when you're asking it to you know analyze your data people want a level of of consistency that we're still not seeing in the AI space and so um there's lots of you know interesting things happening and interesting experiments but you're still relatively isolated. For those reasons, in partner marketing we mainly have three different parties right? We have brands, advertisers um we have the partners, the publishers and then we have the networks. Um, would you say that AI cases or potential AI solutions applications would be something that is similar for all these three parties?
Or would you say this is completely different topics um for each of them? What we are seeing is like when we first started seeing AI getting used you know fragmentedly in production um, all of our minds turned to automate automation possibilities and what that you know practically means is people look at their wage bill and and and and and and and and and and and and and and and and and wonder whether they can make those wages, that wage bill, smaller by making you know their workforce more inefficient. Or sorry, but if you look at the the wage bills for affiliate marketing, you know most you know condensed wage bills sit within the big agencies and the networks, and so I think a lot of those folks are looking at, you know, internal efficiencies, how to, you know, how to get their staff to spend more time growing their affiliate programs, and less time managing their affiliate programs.
You know, we've all we've worked in this industry for long enough to know that there's a there's a million and one tiny jobs to do on any affiliate program, which is where like the efficiency, like it's difficult to scale this, this program or this, this, this area of marketing. And so yeah, all of those folks with the big wage bills are concentrating on internal stuff. Obviously, publishers are looking at data insights and content creation, they're much less concerned with wage bills, and they're much more concerned with traffic. And so I think they'll, they're looking at it from a slightly different angle. Same on the other side, I guess, right, it's very much linked to their own business and how they work.
And then again, probably about efficiencies, pulling reports, pulling data, doing analysis, looking into what kind of partners would fit best for me. Would that be the use cases for brands? Yeah, similar for brands, the problem you face as an affiliate marketeer at a brand is trying to figure out how to do that. And so I think it's very much linked to their own business and that's, you know, the challenge. And so I think for, you know, AI to have any impact on, on your data insight, you clearly need to pass some of your data to an AI model. And if you're the affiliate marketeer at a large brand, there's quite rightly, hesitation to pass too much transactional data into these models.
And so therefore, the, you know, the affiliate marketer, from what I've seen, has kind of really struggled to unleash AI on their data insights. Data set mainly from a privacy point of view when you like partner marketing is probably the channel with most people interacting with each other, like when you work with search you work with Google or you maybe work with Bing. When you work with social media, you work with different platforms in partner marketing, you as a brand you have the network and then you have hundreds, maybe even thousands of partners, you're dealing with. I guess that is exactly where the potential for AI could be, but I guess this is probably the reason as well why there is so much complexity to it right?
Yeah, and I think that question it becomes like a societal question, right? Like at what point are we happy to chat with something that we either know's a machine or someone's maybe not telling you. You're chatting to a chatbot, and like... And it'll be interesting, you know companies like Trade Doubler, you can scale the certainly the long tail of of your clients if you can create mass efficiency by having you know AI account managers. Like the cultural impact of AI account management on society, I think will be super interesting over the next three to five years and... And what we're Happy to chat with and what we're not happy to chat with and and maybe my hope is that we can chat with AI systems about like repetitive admin tasks and we will chat with our strategic humans about more growth-related, strategic things.
Um but yeah it'll be interesting to see how when going back to what you said said before, especially about the wage bills or just now about admin tasks, support tasks, being efficient, managing the long tail. Do you think that with AI there will be a focus mainly on efficiency or on growth solutions as well? Is it more about automation, getting things done easier, quicker, more efficiently, or is there an opportunity as well to scale, scale, grow businesses? I think that will be largely dependent on the models that are created by these frontier models, like the Anthropics, the Open AIs, the Googles of the world. Right now, those models are very good at parroting stuff back to you.
If you've trained the model on how to use Trade Doubler and how to validate sales, and how to get your invoice, and it's very easy for them to parrot that data back to you. What those models can't do right now is any significant kind of chain of thought or strategic thinking. You could pass it, and we've experimented with this; you can pass it playbooks on how to run an affiliate program, but what the models will do right now is just parrot those playbooks back to you. I think what a lot of the model builders right now are publicly working on is kind of more chain of thought, strategic, much kind of richer. Right now, the intelligence of large language models are at, you know, sixth form, first year of university level.
And again, you can train that to do, if you've trained it to do that, you can train it to do that. And if you've trained it to do a specific job, they will, they will probably do that job fairly consistently. And you can do those where they're not what these models aren't at the same level that, you know, your senior account directors are that you're probably using to drive the strategic thoughts on your affiliate program. And the, you know, everyone thinks that, you know, longer training times and more complicated models, means that we'll, we'll kind of move up the intellectual scale to allow these models to give more strategic input onto accounts. But right now, they're just no, regardless of what Sam Altman says, they're not powerful enough to you could maybe use it to identify gaps or something like that, right?
You can run gap. Yeah. But you could have run gaps 10 years ago with machine learning models. And then again, that will probably go rather into the efficiency side again, then. Yeah, yeah, yeah, yeah. And like, yeah, so that, that the way the way we looked at the way that we've, we've been building things is, there's actually there's this temptation, we've got this new set of things to play with, as technologists, I've got these large language models, I can ask them things, and they give me responses. But actually, what we found over and over again, that, you know, like traditional machine learning, technology, techniques are much better at things like gap analysis, than these modern kind of, you know, generative AI solutions.
And so I think, yeah, you know, chat GPT launching and giving us an API kind of sparked the imagination of everyone. But that's, that's led, in some ways back to rethinking the things that we we have been building for, for decades. Now, you mentioned, chat applications that, I guess, are working, right, I've, I've used some of them as well. But they are not as widely adopted or, or likely to be used by the users, then in the end, where else do you think is something to do? So, yeah, interesting-that chat front-end, like we all thought that, I don't know, we'd be booking our virtual content vacations through a chat front-end, like we're not we're not seeing that and I don't like, I don't see me doing that yet.
I think as like, as memory within these large language models get better, I do like, I do see a world where you know, I can feed these models all of my previous vacations and it has the context of that and what I like and what I don't like and what my kids like and what my wife likes and and all of my previous decisions, and at that point, it's probably much better than me logging into to booking. Come and kind of selecting my holidays, but but now it just kind of doesn't have enough context to be to be super useful. Is there any use cases yet or any kind of best practices on the network side in part in the partner marketing industry that you could share with us?
I think some of the people that are doing so... I think the things that are working well now you have to, you have to think about what's good and bad about large language models right now and bake that into your use case. And if you understand that the the large language models create efficiency but are inconsistent then you have to use that to your advantage. And so like a lot of stuff that we've been doing affiliate AI and other People are experimenting with it like first-round weekly reports, first-round monthly reports. Reports that you're going to get a senior account manager to check, but you know, running the data, looking for red flags, looking for who's up and who's down, and passing that data to a senior account manager to uh, to check over, I think we're seeing you know a lot of success and efficiency there, and because that that handles the um, the inconsistency problem by adding a human layer on top of the outputs.
What we're also seeing on the other end of the spectrum is like outbound sales; there's a whole world of sales, outbound sales to, to new Clients for the networks, but also new publisher relationships, and a lot of people are using 'like' there's a whole new wave of um, effectively sales prospecting tools that are taking on um, the data about your specific value, and training large language models to send hyper-specific and much more performant outbound models marketing that um, then you you could do before with you know we all, we all get a thousand automated inbounds a day, and so there's a lot of people you're raising a lot of money off the back of um, of revenue that they've grown in the proof that you know you can use large language models on like mass prospecting.
Where candidly, like you're okay at messing up one in 20 times because your your aim there is scale again in the middle would I use would I use that first tool to send reports directly to Trader's biggest accounts no it's too risky I wouldn't do that. Like, would I use that second tool to prospect if I was just going after a strategic list of 30 accounts no it's too risky. But you kind of have to bake in the known problems on either side as you mentioned already in the beginning I guess the the basis of everything is the data that is available and can be used for any kind of AI models or solutions. How, how is that to be tackled is That's where the challenges are, yeah I think you know every single company on earth has tried to clean up their data every couple of years, I think that's an ongoing process, you know.
Most people, um, have some sort of data lake use data bricks or one of the commoditized data platforms, um, available in all the cloud providers. So, yeah, I think that's a something that everyone needs to to build on a a clean bed. We're also, you know, large language models right now are not that great with structured data, much better at unstructured data, but you obviously you still need super clean first-level data to then turn it into unstructured. Data and so, yeah, I think. But I guess this is a little bit where the trick is, because we've been working so long and with such a focus on structuring data and storing it accordingly. Now getting this structure out of the data again is the challenge, right?
Yeah, yeah, and I think there will be...actually, and treason Horwitz um put out a call to start companies, and we will invest in you, like this wave of, you know, selling companies like Trade Doubler tools to take you know their structured data and turn it into unstructured data. Because, you know, every company on earth that sits on a on a pool of interesting data is going through the same problems and so yeah that like you'll remember that the tools in ai just don't really exist yet we've only had access to to gpt3 3 . Five APIs for two years, and so like the toolkit that we take for for granted building on top of like all the things that you can just put a credit card into AWS and get the toolkit out to do you know whatever technical job you need to do.
Most of that baseline toolkit doesn't exist in AI right now, and that includes, you know, the structured to unstructured work. Besides this kind of thing, every everybody's speaking about AI; some even say they have some kind of AI structure. What is your experience when working with some of these companies about like being really ready to do something and the willingness internally um to work with AI challenge statuses and trying something new? Is that as open as you would think that is? Are people prepared for that? Um, what I have seen is this space is moving so quickly technically you need to be prepared culturally and tooling wise to move at a at a fast speed. It's very what you can't do in AI is write a 12-month roadmap and like hope to stick to it because next week you're going to have you know new techniques and new models and new ways of doing things, and you're going to have to do something different.
lot of thinking and new cultural changes and so for me the most important thing if you are building with an engineering team is you know having the right culture of experimenting and moving quickly and like checking one of the one of the biggest mistakes that affiliate ai made was to presume that people wanted to chat with their data and i thought they wanted to chat with their data they told me they wanted to chat with their data but right now no one actually wants to chat with their data and so if you are if you are building new things that that you don't have a playbook from what people have done before like you need to move quickly and experiment to prove that it's like a real thing it's very easy in ai to lose 12 months building a thing and it's very easy to lose 12 months building a thing and it's very easy to
lose 12 months building a thing that that you realize you know doesn't add enough value and so i think my advice to anyone trying to build stuff is is that it you know you have to have the cultural setup there of experimenting and validating that your experiments have have moved the number that you're trying to move rather than just you know playing around with these new technologies or or trying to plan too far ahead when there's so many Unknowns right now, and I guess besides this culture, I guess you need dedicated people right so you need people that focus and concentrate solely on this um obviously coming with an investment right yeah maybe I think I think this will be a toolkit that all engineers use over the next couple of years and so I would you know you need you probably need to you probably need a couple of people who have at least played with with some AI tooling and hopefully shipped something but more importantly kind of excited about it and then you need to you know use them to get the rest of your engineering team you know interested and like adding these large language models as a tool in their already existing toolkit i don't think i think you know a lot of times i think sometimes we see ai as a hammer looking for a nail and i think that's the wrong way of building products i think you should be like you know we want to drive well we want to drive more gross profit for trade doubler and our leading indicators to driving that gross profit are these three things we need to be building products that move those leading indicators that then drive the profit at the end i don't think we should be marauding around with these things we need to be building these new Toys that we have, um, trying to to do things that solve problems that don't necessarily need to be solved. Now, got me interested, so what would be your advice on on how should I get started? Um, I have nothing; I would be just think about starting now. Yeah, I would be rigorous on um understanding what numbers you want to move and so I would you know, have my my main aim probably, you know, revenue or GP or or like whatever that I would hypothesize on the leading indicators that run up to that eventual goal, and so that might be, you know, more advertisers, more publishers, more partnerships for those publishers. Like, how do we, how do we get This two-sided marketplace churning better, I would make sure I would have the tools and rather I think that. Excuse me, both the data and I wanted to providers will ship something new and so you know when anthropic ship their new models on a Monday morning, I want an excited engineering team with very clear measurable things that they can experiment against to drive those leading indicators forward, that eventually you know spills more more profit or revenue out the other side. Before starting that, I need to get my prerequisites in place right, like the data, I can really deal with um the applications API connections um. Yeah, yeah, what else is there? You're gonna need like this: there's three or four model guys, like you're gonna need to you know they'll have standard contracts. You know these these companies are so overrun with people wanting to do business with them, you're not going to be able to redline that contract.
But you need to get your security team happy with with doing business with these people. Um, like for example: you can interact directly with the Open AI API, or you can sign an enterprise deal with them that gives you more um, more kind of privacy over your data; or you can pull largely the same models with Azure. like you kind of you need to get your ducks in a row to make sure your legal and compliance teams are happy with the data and you can do that with the open ai api and you can do that with the open ai to put those tools in the in the hands of right because you don't
want to wake up in the morning and you know anthropic have shipped this incredible model and now you've got to wait three months for it to get through legal if somebody tells you to stop again yeah yeah yeah but yeah you're like you you need to get the building blocks in place you need like clean data you need a cultural culture of experimentation you need the toolkits to experiment If you're engineers want to split-test the chat bot against the non-chat bot and they don't have the uh, the data to see whether that moves any numbers, you're just kind of shooting in the dark. Now, you're the founder of Affiliate. AI, um, I think you set that up some two years ago, right? Yeah, a couple of years ago, just like yes.
What triggered you, so I've been pulling on the thread in my like my brain's always trying to start a company; I managed to suppress it most of the time I go for a long run, I'm writing a business plan in the back of my head for... I don't know, a coffee shop or like a new software company or this is kind of how my brain works. Um, and I'd been pulling the thread on pulling together affiliate network data and doing something interesting on top of it. I think affiliate marketing sits on so much rich data, and like outside of of paying people out on it and doing a few arbitrary conversion rate metrics... I don't think we're we're driving enough value from that data set, and so I was thinking about like is there a similar web for affiliate marketing?
Like what can we do to kind of build something on top of this data set? And then you know the Open AI APIs launched, and then all of a sudden I had like this new toolkit to play with, and we started You know, I picked up a couple of part-time engineers and we started playing with like how do we, and you're obviously affiliate networks have public APIs like Trade Doubler. They have got a very good API with the right permissions from an advertiser or a publisher, I can ingest their transactional data without them needing to do any engineering work at all, and so I kind of had what I thought was there's these two magic things: I've got this clean data source that I can pull out.
All I need is a non-technical person to log into Trade Doubler, copy and paste an API key, and then I've got you know hundreds of millions of transactions that I Can I look at, and then I kind of have this new toolkit of AI, you know, APIs to play with, and then I thought, 'Hang the second that we could, we could build something magical here,' um, and then I spent, you know, three months buying everyone in affiliate marketing a coffee. I've been working in affiliate, you know, since 2005, and so I had plenty of people who I could buy coffees for, um, and everyone, everyone was talking to what's effectively a take-rate problem, you know, there's largely commoditized technologies in affiliate marketing which leads to downward pressure on margin.
I thought these AI technologies could probably you Know, create efficiencies within the workforce. You know, I was an account manager once, and I know the inefficiencies that are running affiliate programs. You know, that took me a long time to figure out how to do that, and I think that's one of the things that I've been working on for a long time. And I think that's one of the things that toolkit for affiliate marketers hasn't really changed since I was an account manager at DGM in 2005. You're still logging in and clicking things, and using Excel, and and so, I kind of i thought at a macro level, you know, there was something that we could do here to to alleviate that downward pressure. On margin with this new toolkit, um so yeah, we started building and experimenting.
Um, built some software, um, did a lot of work on it and I think that's one of the things that I've been working on for a long time. And I think that's one of the things that I've been working on for a long time-a whole bunch of consultancy, um, a lot of people, a lot of you know networks and agencies sit on you know these big wage lines and these big, you know, troves of of proprietary data. And I think there's a lot of exciting things we can do independently and within the walls of of those companies. Now, you say you work in partner marketing since 2005 almost. Like me, 2007 um, partner marketing is anyway I would say one of the most innovative um digital marketing channels um that are out there.
Would you say that AI is um has the potential to drive this innovation and partner marketing even further? Yeah, very much so. I think it's it's the reason that is partner marketing is so innovative that's largely on the publisher end, yeah, and I think that's driven by the like the purity of the CPA model and like when and it you know it's different now but when I first started in partner marketing okay no one really asked too many questions about how you were getting your traffic and your sales. You know, that clearly led to a bunch of fraud. But what you could do is, you could get accepted onto you know, you could get accepted onto you know, you could get accepted onto.
We were my boss was 19 and I was 25 and you could get accepted onto like the BT affiliate program and start doing business with British Telecom and getting paid hundreds of thousands of pounds a month from this enormous company. Like there's no other channel on earth that would allow you know, that type of relationship. And I think that really spurred the uh, the innovation certainly on the publisher side. I'd argue that it's probably A partner marketing is probably also the least efficient channel in online marketing and, and you know some of that's a feature like it is partner marketing; it's relationship-driven, it's you know, you're having partnerships there's there's a lot of exchanges outside of of transactions and money um but you know it does lead to you you know, a whole bunch of you know admin tasks in the middle that up to now have been very difficult to automate because they were they were nuanced; but also like it's it's death by a thousand paper cuts right?
You can't you can't automate each one because there's a thousand different tasks. I do think, like, we're seeing um tools from Anthropic where it can take over your home screen of your computer and start doing things. I do think there's an interesting world where we can train AI models to do some of those small repetitive tasks much more efficiently than we could train automation on before, um, which you know could unleash this you know a second wave of of innovation in partner marketing which has been hampered by you know a lot of the things that the several years have gone on and like all these video BI projects and all that, you know, especially uh commerce space, where people are Glenn Conley. And a lot of people like doing mining data infrastructure stuff where they're like putting together they're illegal police all kinds of things, and there are meat sites if you're looking for the size of offices and stuff inside their humans to build a piece of Internet and put hundreds of verbatim über every single device out there. I don't think that's something that's going to save people; it looks like there's a lot of debate, it just looks like I'm okay, I'm okay. Organizers charge that because of whether to just pay and pay things you can't get away from 100 at least, I don't know who has this sort of everybody's arguing that. Could be vouchers, for example, because you don't need these websites anymore. You can just like search at the end user, the consumer, can just search for the voucher they need as you said before or or book their vacation um in a customized way. How do you what do you think that will do to the affiliate network in itself so the the industry the the partner, yeah yeah honestly I don't know like my two years ago if you'd asked me I'd tell you that chat was the new front end for the internet and we would we wouldn't be clicking on blue links in Google anymore and we'd be chatting with all of our data um obviously Perplexity are doing very well definitely worth playing around with that they're much better at at citing where they get data from then um then certainly open air um but yeah i think that like chat chat is a front end which is it's the premise right like if we're you know the theory of of coupon sites losing sales is predicated on you wanting to chat about a purchase decision and getting that data straight from the chat as we see very little empirical evidence that that is how people want to interact with websites right now like the ways we want to chat are very the things we want to chat with are very different like they're much more in-depth tasks than um then you know coupon retrieval um so yeah i don't know what what's what's your view what will happen next now when you look into the next maybe two years or so what will be the main trends what what will be next i think the step change the next step change that we will see is i think increasing intellect or levels of intelligence at a model level will unlock a lot more strategic jobs that the llms just kind of aren't equipped to deal with right now i think that becomes super interesting um i think the accuracy a lot you've got To remember these, a lot of models up to now, we don't really know why they do the things that they do or how they do them.
There's a whole wave of of work that's happening within the large language models to to understand the neural pathways and understand why, like, a lot of the problems we see with accuracy are you know difficult to solve because we don't know how they came to the answer that they they came to, and so I think over the next 6-12 months there'll be a greater push for accuracy within the uh the responses which which you know opens up a whole world of use cases as we spoke about earlier, that AI just can't touch right. now um and then there's the memory bit right like if we the more comfortable we get with with interacting with AI models the more likely i am to pass it my history of all of my family holidays so it can do a better job at creating your own personal assistance exactly yeah yeah and like yeah like i think that could be incredible right like if you if if you get the accuracy and the memory to hand over your calendar and a lot of your inbox um to to an ai assistant like the um yeah the step change in in productivity could be astronomical okay thank you so much rob what i take away now is there seems to be incredibly much potential for us especially in partner marketing both on the efficiency side as well as on the innovation side so us as an industry so us as an industry seems to be prone um to work with ai to stay as innovative as we are and make a few huge steps forwards right that's basically my takeaway from from our conversation now yeah yeah uh exactly and like i think you a lot of people have decisions to make whether to to experiment or to sit it out and wait for you know the use cases to be proven um and i think you know a lot of people have that's probably a decision that that that trade doubler are making on a daily basis like how but it's on us how heavy to invest in yeah yeah but i do like economic theory like if if you can if you can trade in a dramatically more efficient way than your competitors like the economic value is is astron astronomical if you're if you're an affiliate network and your cost of service is higher than your competitors then you're not going to be able to trade in a you know anyone that took one class in economics will extrapolate that line to what it means for for your business and the things that you can reinvest in for for innovation true at the end now i have three a little bit more personal final questions i like to ask i ask every guest what is the Best book I've ever read, um, Kitchen Confidential by Anthony Bourdain is my favorite book of all time. I'm re-reading that right now, what is that so... uh, Anthony Bourdain was a very famous American chef. He never he was never particularly successful chef-wise, but he kind of wrote this seminal book on you know, like eating and cooking and going to restaurants and you know, you know, you know, you know. He has these amazing stories about, he's a very he was a very articulate man. Um, about his first oyster and how you know you shouldn't order fish in a restaurant on a Monday, and it's it's it's a it's a magical book that I've probably read 10 times in my life and I'm currently rereading that's good, I I will get that one, yeah, I'll send it to you, thank you, and um what uh which tool or app, you say um, you absolutely can't live with without of course um, I'm, I'm using Anthropic, Anthropic desktop a lot right now as like a sounding board and as as a researcher, um, I think certainly from an AI tool point of view
it's $ 18 a month, like it's ridiculous value for the amount of time you can spend, and I think you know if you're in any way interested in AI, the only way to really get your get a grip on it is by playing with these tools, so I would pay your 18 for Anthropic. Pro and install the desktop app, and I have Genie, but I will yeah, I will try, yeah, I will give that a try as well.
Good, and then, lastly, if you were not working with AI, what would you do? Starting some other harebrained company again, I mean, I know any given day I'm starting a coffee shop in my head or another software company or like I think entrepreneur yeah, I mean if for me it's like a creative endeavor to like build new things, um, which excites me. Thank you so much Rob, great talk, uh, thank you for sharing all these insights with me, thank you, thanks for having me, thank you very much for listening to this episode of The Partner Marketing. podcast stay tuned for the next session you find us wherever you get your podcasts this podcast is made possible by is produced by TLDR Studios.